Engineering the Experience with Sensorial Brand Engineering at DCA
DCA’s approach to Sensorial Brand Engineering (SBE) forges efficient links between qualitative and quantitative data to fulfil complex product requirements.
Let’s start by considering a product you might be aware of - the Lynx/Axe antiperspirant pack. This ubiquitous product - almost 10 billion units have been sold since its launch in 2014 - was engineered by DCA in partnership with Unilever’s Deodorants Global Design Centre in Leeds. Interacting with this product is a multi-sensory experience – think about the ‘tssssss’ as the button is pressed, the cooling effect of the spray on skin and the aroma of the scent. To the commonly recognised five senses, we can add the kinaesthetic sense, defined as the cognitive and physical reaction to movement. This kicks in as the user twists the cap through 90° to raise the button and prepare the pack for use.
Whether this holistic sensory experience falls short of, meets, or exceeds the user’s expectations is a primary driver for customer satisfaction. Appropriately matching the sensory experience of a product to its associated brand – Sensorial Brand Engineering (SBE) - is critical to delivering customer satisfaction and building a cohesive brand identity.
Clearly, delivering customer satisfaction requires detailed consideration of a huge variety of competing factors. This is evident in the model shown below which was introduced in our article published on the 14th March 2016 - What Is the Relationship Between Product Quality and Customer Satisfaction?
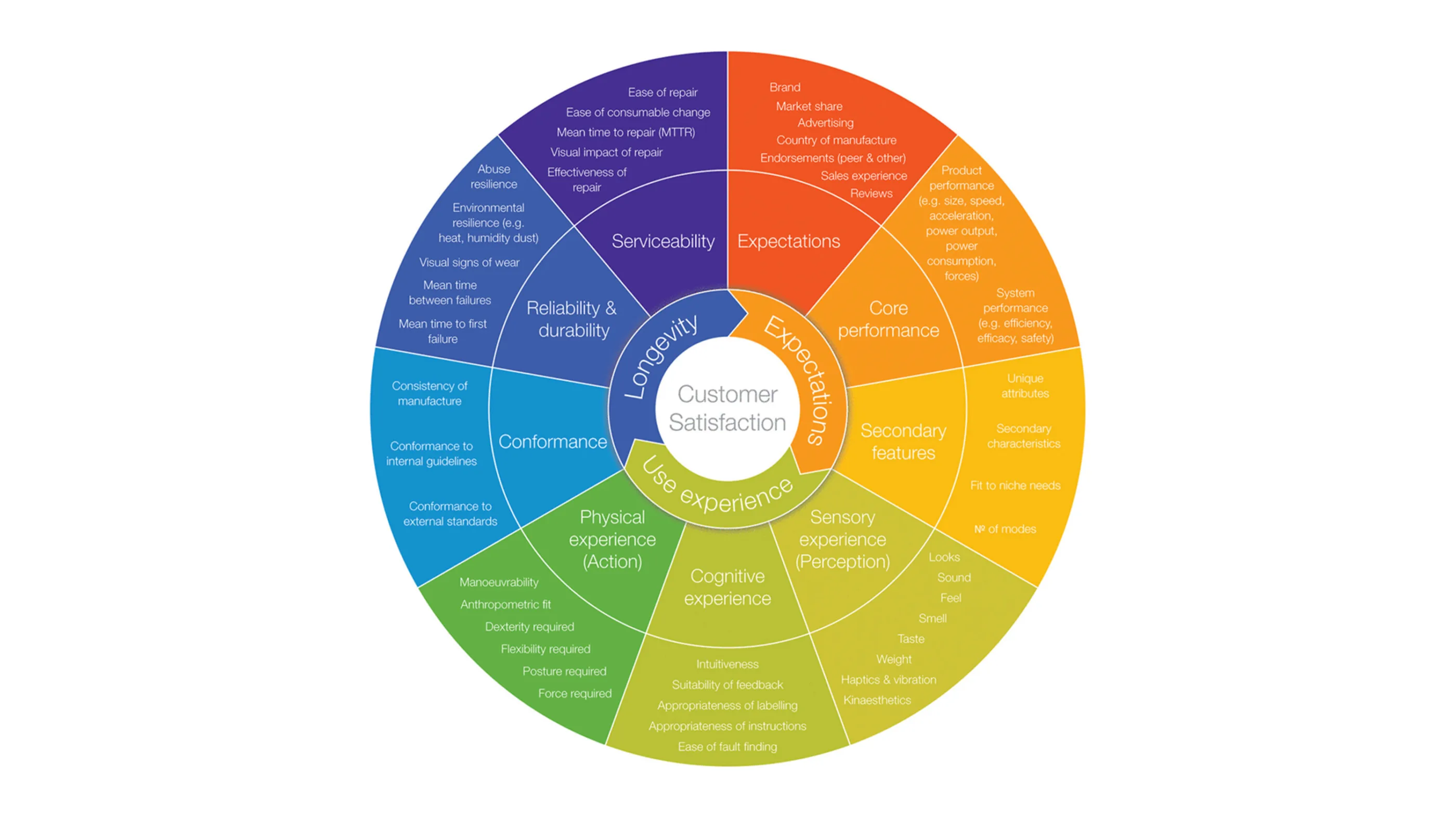
Sensorial Brand Engineering forms a critical part of the Sensory Experience (Perception) sector of this model. However, it is often carried out without scientific rigour. This means that the development team can be targeting the wrong combination of user experiences. Alternatively, even when the correct experiences have been identified, multiple trial and error cycles are required to achieve the targets. In short, correctly deploying SBE is a highly demanding task.
SBE challenges
Sensorial Brand Engineering provides a unique challenge, chiefly because it requires detailed quantitative and qualitative input from several diverse disciplines – primarily mechanical engineering, design research, human factors/usability and industrial design.
Clearly, delivering SBE solutions requires experts within each discipline with access to the necessary resources. However, on its own, this is not enough - the diversity and range of the input required creates numerous opportunities for inefficiency and miscommunication. Co-location of these areas of expertise under one roof, as at DCA, is a critical first step in driving an efficient process. Add to this DCA’s recently developed process to integrate and align the input from each discipline, and you have the potential for efficient delivery of truly world-class SBE solutions.
The SBE process at DCA
The flow diagram below shows how we take a single input – the desired Sensorial Brand Language (SBL) for the product – and move to a single output – a physical product matching the SBL. The process may be applied either before or after initial prototypes are available and may be used to optimise input to any of the human senses – sight, touch, hearing, smell, taste and kinaesthetic. Of these, the hearing and kinaesthetic senses are most commonly targeted for optimisation.
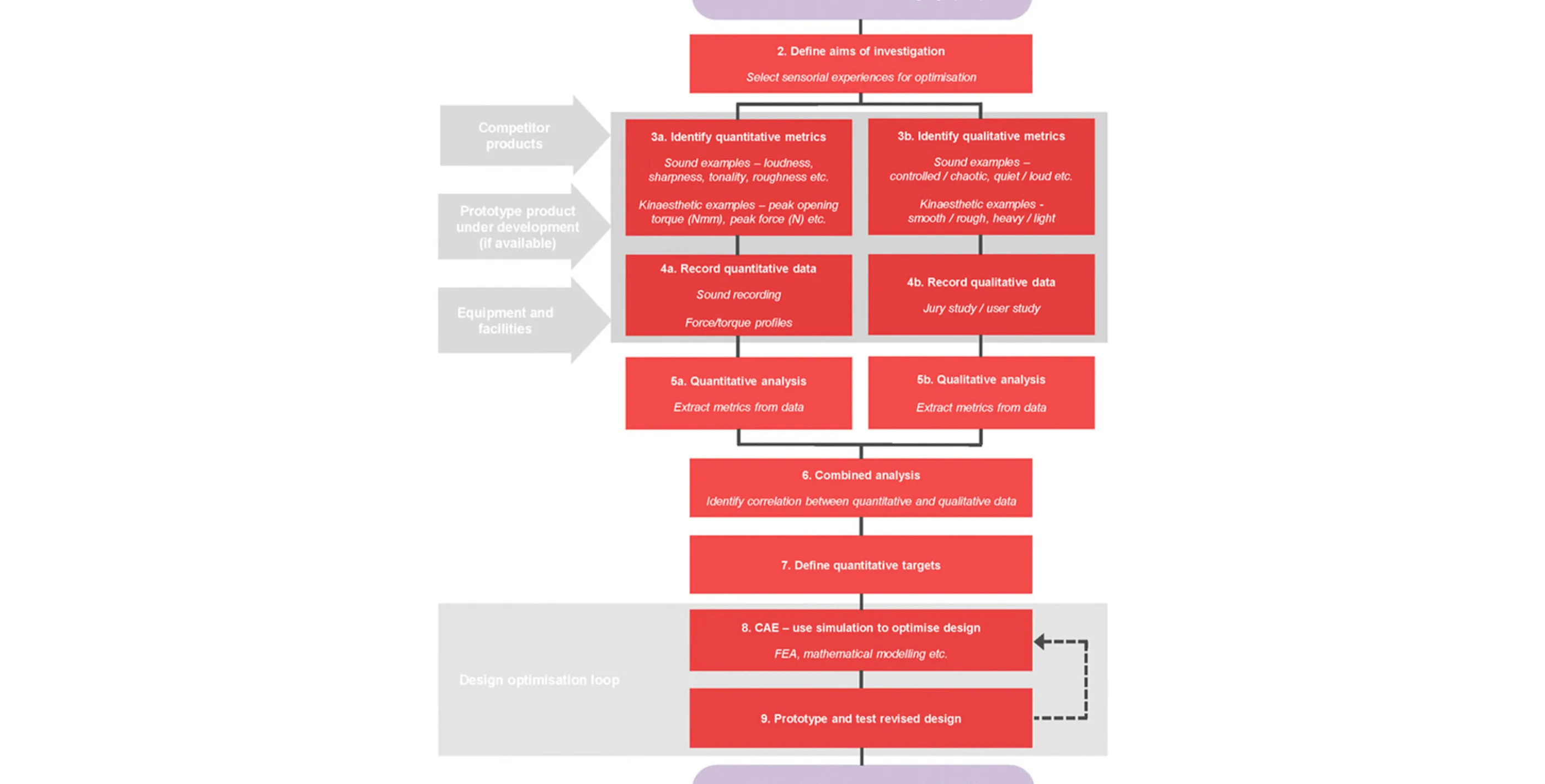
To explain why this process is necessary, imagine that a new hair dryer is in development. Early prototypes have undergone initial testing and received feedback such as ‘it sounds unexpectedly loud’ and ‘it sounds like it lacks power’. This raises a multitude of questions:
- What aspect of the sound is responsible for the feedback - loudness, frequency content or something else?
- What changes to the sound should we be aiming for?
- Which physical features of the prototype are responsible for creating this aspect of the sound and how can we adjust them to make the desired change to the sound?
Even trying to qualitatively describe a sound is difficult - imagine trying to translate this verbal feedback into quantitative technical requirements and design changes without a robust process – a near impossible task, and certainly one that would rely on luck rather than judgement.
DCA’s clearly defined process enables this complex range of multidisciplinary questions to be broken down in a manageable way, facilitating an efficient interchange of information between each specialism.
Stepping through the process
The input to the process is the desired SBL for the product (box 1 in the flow diagram). The desired SBL will likely consist of a set of word pairs, with the target SBL positioned on a sliding scale between the two words (see example below).
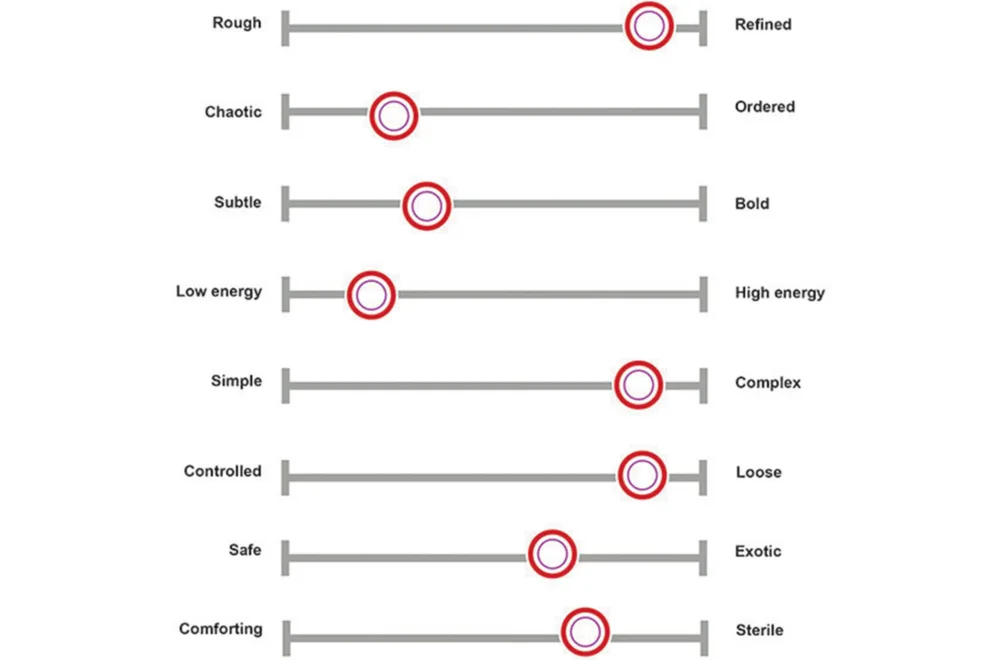
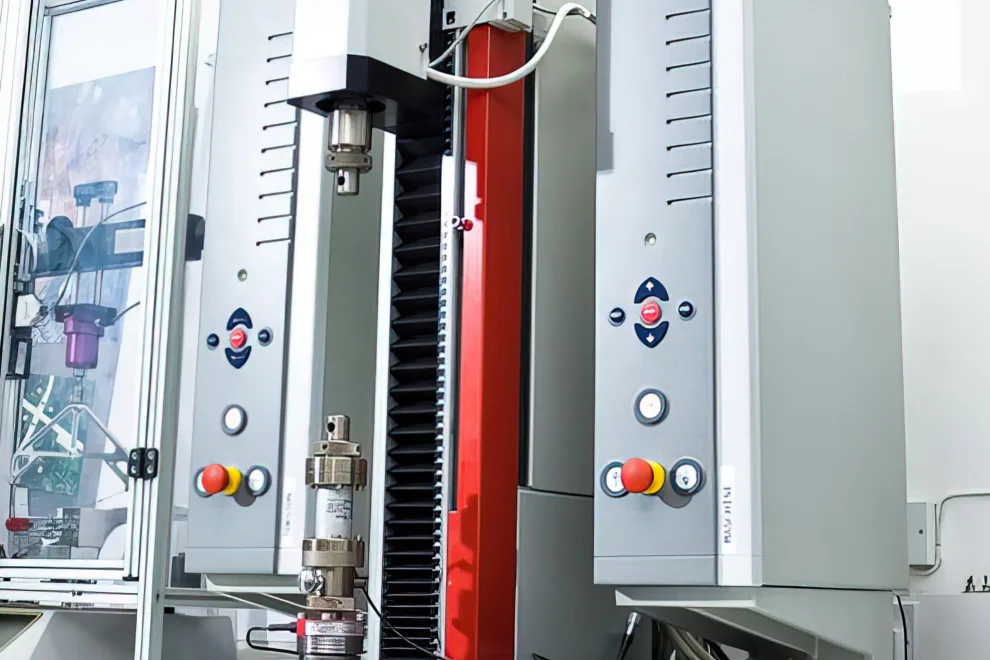
The next step is to clearly define the aims of the investigation (box 2). This means isolating a particular sense for optimisation. Isolation of a single sense is necessary to ensure that the results are not influenced by input from other senses.
The investigation then splits into the qualitative and quantitative paths which run in parallel. On the quantitative path (3a), the first task is to identify the critical metrics that best describe the sensorial experience in question. For a study involving sound, there are multiple internationally recognised psychoacoustic metrics such as:
- Loudness
- Roughness
- Sharpness
Selection of the appropriate quantitative metrics for a sound-based study requires in-depth specialist knowledge of acoustics. Many of the standardised metrics originate from automotive, aviation or health and safety applications, and there are some limitations on their use for product sound quality evaluation. If required, we are able to use our multidisciplinary experience to ‘tune’ these standardised metrics to better suit the particular needs of the study.
For a study involving the kinaesthetic sense, such as the twisting cap on the Lynx/Axe pack, then the quantitative metrics might be:
- Peak opening torque
- Maximum deviation of the steady-state opening torque from the mean value - an indicator of the smoothness of the motion.
Meanwhile, on the qualitative path, a similar process of metric definition occurs (3b). Whilst there are many evaluation methods for subjective testing, we find the most appropriate to be the ‘semantic differential’ method as this allows us to evaluate multiple attributes at once. Simply put, this involves using pairs of words at opposite ends of a scale, which participants use to evaluate the sensory attributes of a product. Selection of suitable word pairs is an involved process – the words must be product-specific and meaningful to non-technical persons without carrying an excessively strong positive or negative connotation. For example, for a sound-based study on hairdryers, the word pairs powerful-powerless and quiet-loud may be used.
Next, we move into the data acquisition stage. On the quantitative path (4a), the process involves testing each product using specialised equipment. For a kinaesthetic investigation, DCA’s bespoke Zwick/Roell Z0.5 test machine (shown above) allows force and torque data to be acquired simultaneously. This machine was used extensively in the development of the Lynx/Axe actuator. For a sound-based investigation, our semi-anechoic chamber allows reliable, repeatable sound recording.
On the qualitative path (4b) a user study is conducted. In this study, participants are asked to evaluate a range of prototypes and competitor devices using the word pairs chosen earlier. We may also ask participants to rank the prototypes in order based on additional attributes specific to the investigation such as quality or annoyance. These activities are followed by a semi-structured interview to dive into the reasons why participants favoured some prototypes over others and learn how we could improve them.
As mentioned earlier, it is critical that the users are responding only to the sensorial aspect that is the subject of the investigation. For a sound-based investigation, this would first require the sound produced by the products to be recorded in our semi-anechoic chamber using a binaural microphone. This interesting piece of equipment uses a pair of microphones fitted within an artificial ear structure, allowing stereo recording of the sound actually reaching the eardrum. The recordings would then be played back to the members of the user study using stereo headphones, allowing an uncannily accurate replication of the aural experience of using the product - but isolated from the muddying influence of the physical products themselves.
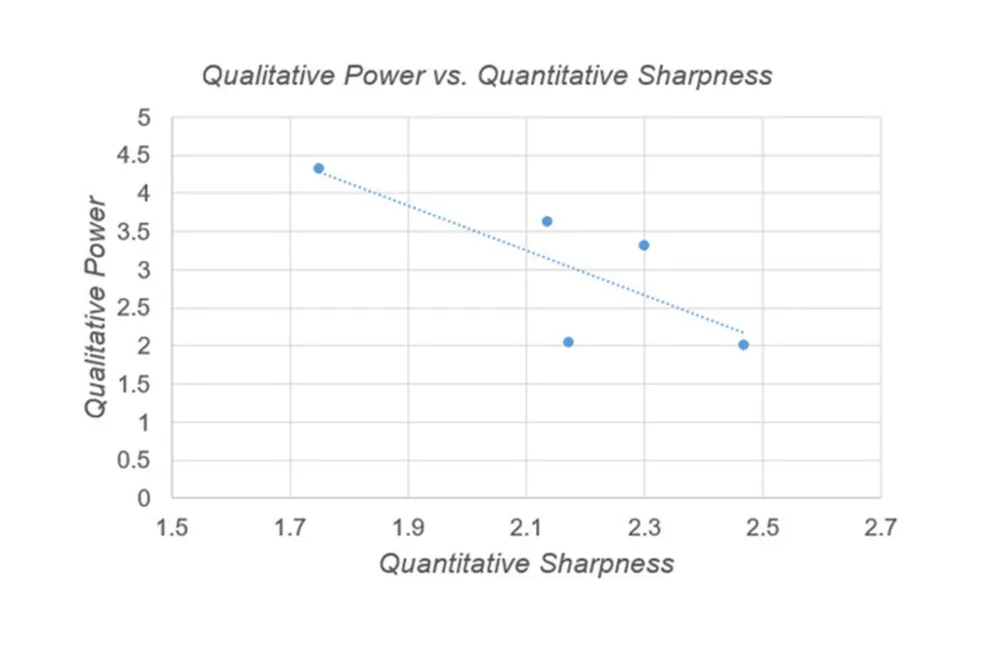
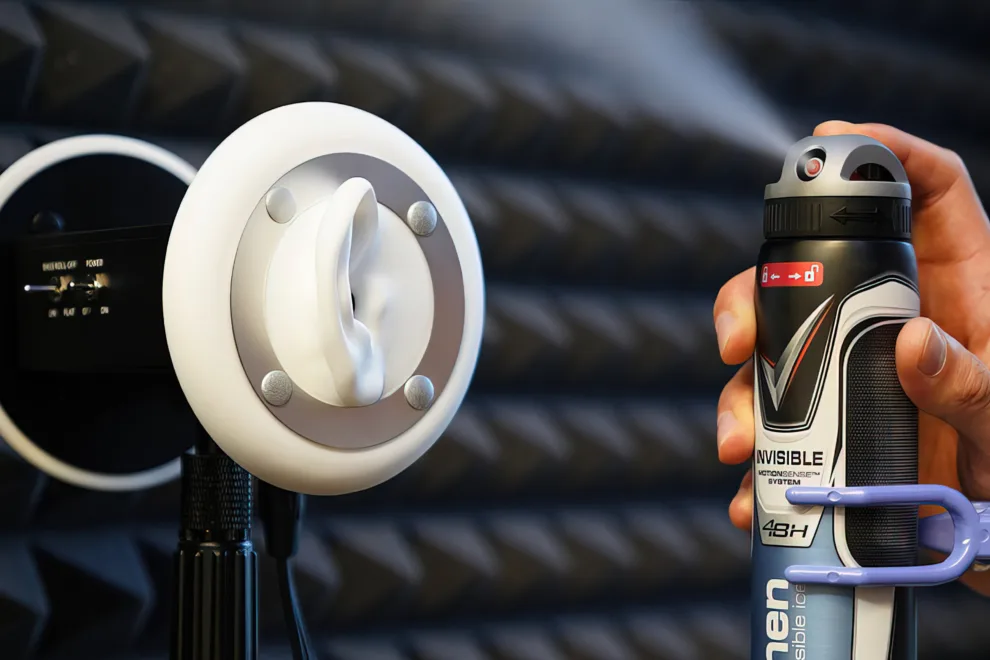
Next, we enter the quantitative analysis stage (5a). Here, the type and quantity of data recorded will influence the processing technique. For a sound-based investigation, specialist sensorial metric analysis software packages exist, but these are limited to analysis of standardised metrics. As mentioned earlier, it is often important to ‘tune’ the metrics for the product in question. We find MATLAB software allows rapid and effective processing of sound recordings and extraction of both standardised and ‘tuned’ metrics.
The next step is to bring together the quantitative and qualitative paths in a combined analysis (6). This involves using a multivariable statistical regression technique to look for statistically significant correlations between the quantitative and qualitative data. For example, in a recent hairdryer study, we discovered a correlation between the quantitative sharpness of the sound (how high-pitched the sound is), and the qualitative power (how powerful the user perceives the hairdryer to be). In short, the higher-pitched products were perceived as less powerful than their lower-pitched counterparts.
Forging this link between the qualitative and the quantitative is the crux of the whole process. It would be easy to assume that quantitative loudness is the key driver of qualitative power – but our rigorous process showed that quantitative sharpness was actually much more critical – a valuable insight. Without this process, a design team seeking to increase qualitative power might follow the intuitive route and decide to increase quantitative loudness. Not only is there no clear target definition, but the wrong variable is being optimised – a huge inefficiency.
With our process, we can be sure that we are optimising the correct variable – in this case, the sharpness of the sound – and we can also define exactly what frequency we are aiming for, in Hertz. The value added is clear.
Making the right changes
With the quantitative targets clearly defined (7), we can now move into the design optimisation stage, confident that we know exactly what changes are required to match the SBL definition. Typically this will begin with Computer Aided Engineering (CAE) (8), followed by prototyping and testing of the revised design (9).
The Lynx/Axe actuator demonstrates DCA’s considerable experience in this area. In this product, the cap is twisted back and forth by 90° to raise and lower the button, a kinaesthetic experience that was identified as a critical component of the product SBL. The torque profile is largely controlled by a pair of flexible polypropylene ‘fingers’ which are deflected by a profiled track as the cap is rotated.
The two fingers are initially flexed outwards as rotation begins, storing strain energy. The fingers remain flexed through the bulk of the rotation until the cap nears its final position. At this point, the track deviates back inwards, allowing the fingers to return their stored strain energy as a ‘pulse’ of torque that drives the cap home, providing the snappy, precise kinaesthetic feedback required by the SBL.
During development, Finite Element Analysis (FEA) was used extensively to optimise the design of the fingers (8). The key challenge was to maximise the strain energy that could be stored whilst minimising viscoelastic stress relaxation within the fingers. This was achieved by carefully tuning the geometry to evenly distribute the stress and minimise local stress concentrations. Optimising the ratio of tensile and compressive stress within the finger was another part of the puzzle – polymers are generally more resistant to viscoelastic deformation in compression than tension. The addition of stiffening ribs on the rear of the arm (which was subject to compressive stress) provided the desired stress ratio. Moulding considerations also played a large part in the design – avoiding ‘sink’ on the visible faces of the cap limited the maximum wall thickness of each finger.
The FEA allowed the stiffness of the fingers to be derived. This value was then input into a mathematical model, along with track profile, allowing prediction of the resulting torque profile (8). The plot below shows some sample torque plots from development, both measured and calculated.
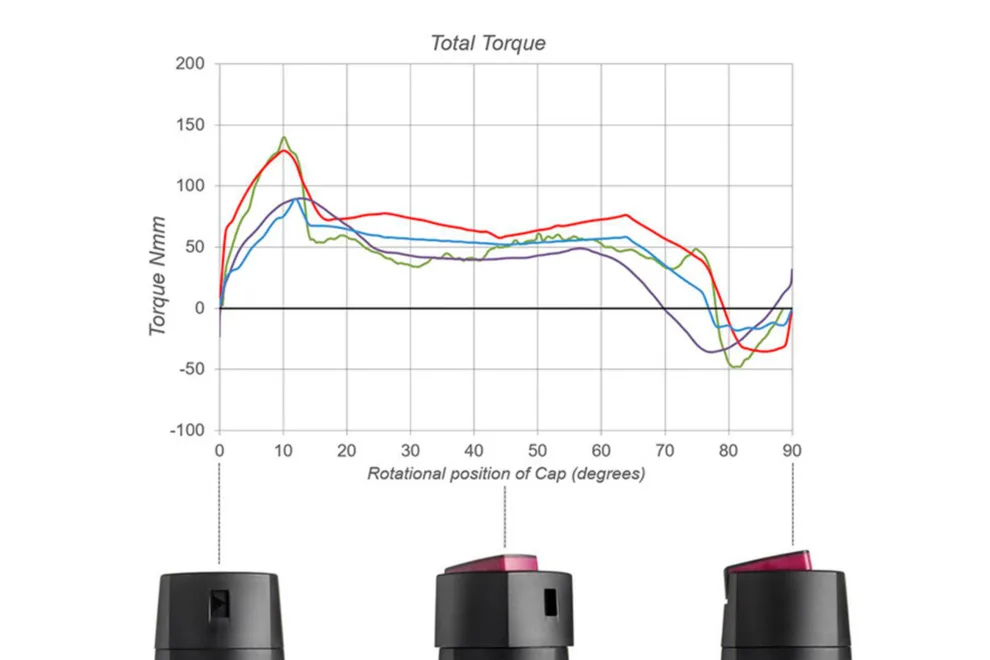
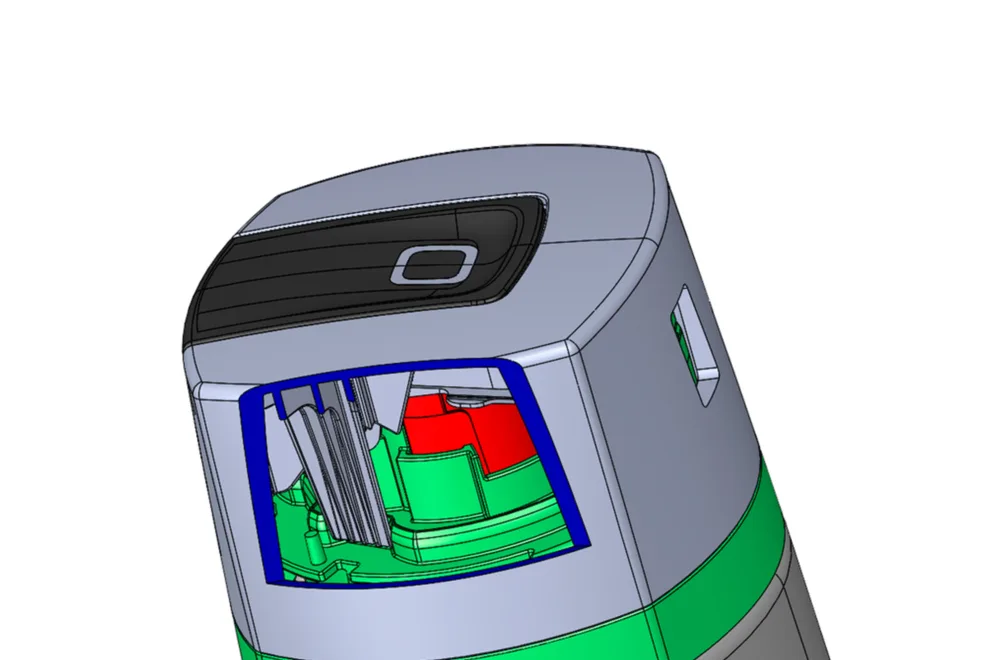
Performance of a physical prototype remains the acid test, and was a crucial part of the development process for the Lynx/Axe actuator (9). However, the FEA and mathematical modelling process reduced the number of time-consuming prototype/test loops to a minimum, allowing efficient delivery of a product perfectly matching the desired SBL (10).
The bigger picture
The significance and power of the SBE process outlined in this article should be self-evident. It efficiently integrates cross-disciplinary input to set out a rigorous, data-driven path from a desired Sensory Brand Language to a product or prototype which meets that SBL – a process too often driven by gut feelings and educated guesswork. Despite this scientific rigour, it is flexible and can be applied to any of the human senses, at any stage of a project.
In short, it ensures that this particularly complex segment of the customer satisfaction model has been efficiently and thoroughly optimised.
Article written by Paul Griffin, Senior Mechanical Engineer and Sophie Sladen, Mechanical Engineer.